Comparing USGS ShakeMap versions
The M 7.1 - 72 km ENE of Namie, Japan earthquake.
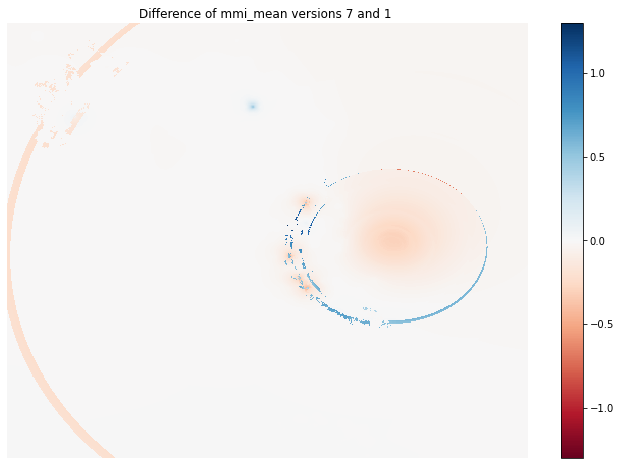
Recently, I came across the question if and how it is possible to download superseded versions of ShakeMaps distributed by the USGS Earthquake Hazards program. This post comprises what I encountered on my first walk through the rich resources offered by the USGS.